NEWS
An energy-efficient semi-supervised approach for on-device photoplethysmogram signal quality assessment
IFH’s research, published in Elsevier Smart Health, has received the best paper award runners-up from IEEE/ACM international conference on Connected Health: Applications, Systems and Engineering Technologies (CHASE)!
- Feli, M., Azimi, I., Anzanpour, A., Rahmani, A.M. and Liljeberg, P., 2023. An energy-efficient semi-supervised approach for on-device photoplethysmogram signal quality assessment. Smart Health, 28, p.100390.
This research introduces an energy-efficient machine learning technique designed for on-device assessment of photoplethysmogram (PPG) signal quality. The study conducts a comprehensive exploration of PPG signal morphology with the goal of pinpointing precise and lightweight features that can ensure dependable PPG readings. Through their semi-supervised learning approach, which relies on robust similarity measures, the research effectively distinguishes trustworthy PPG signals. Rigorous testing on embedded devices corroborates the effectiveness of this approach, revealing a remarkable balance between accuracy, energy efficiency, and latency when compared to contemporary methodologies. These findings hold significant promise in bolstering the reliability of wearable devices.
More detail can be found here.
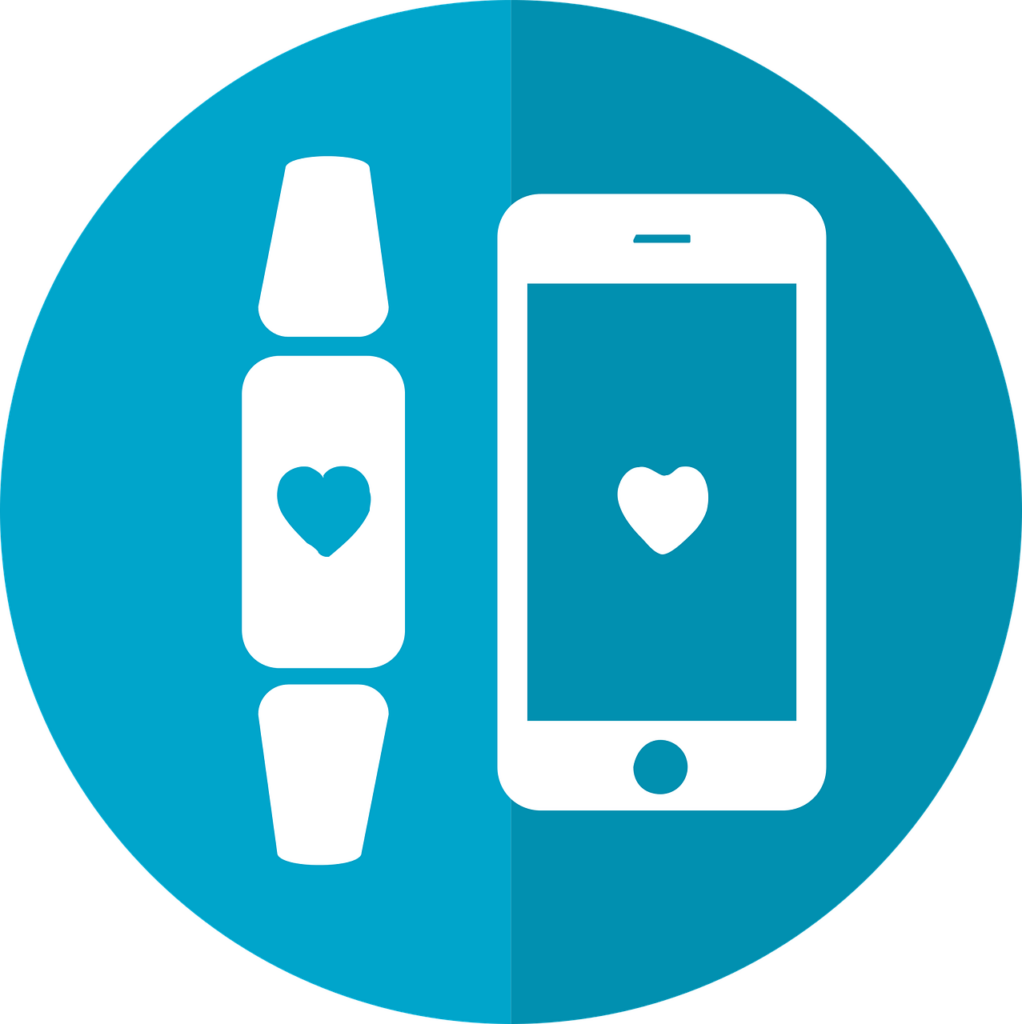